Q&A from the custom patent classifier webinar
We have received a lot of questions relating to the custom patent classifier. As many of them could be of general interest, we wanted to attempt to answer some of the more common ones here.
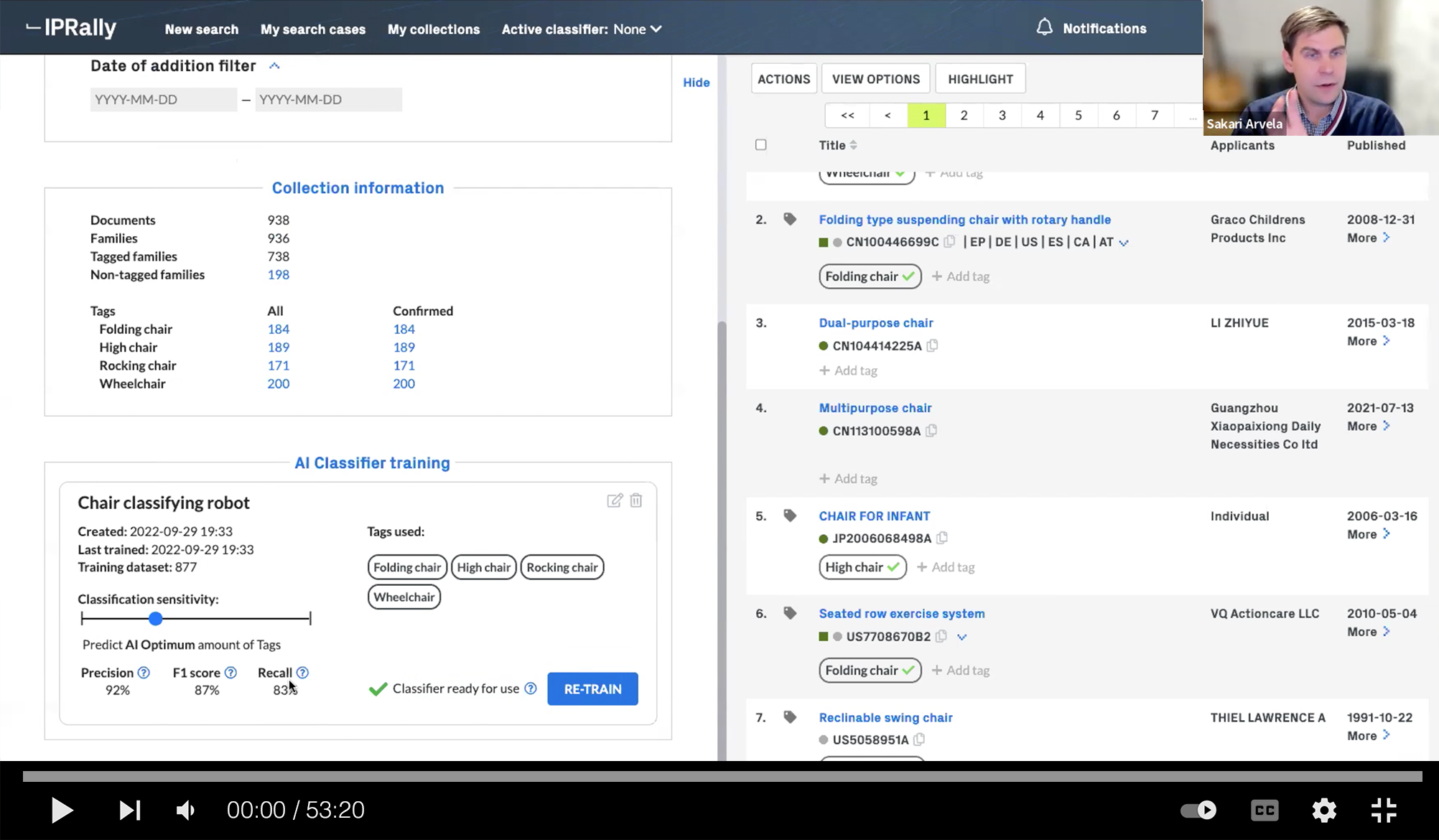
We are genuinely overwhelmed by the interest in our newest development, the custom patent classifier module, and the huge turnout for our launch webinar. We suspected that we were solving some real challenges with this adaptation of our graph AI, and we are now more convinced than ever.
We have received a lot of questions, both during and after the webinar. As many of them could be of general interest, we wanted to attempt to answer some of the more common ones here. So, in no particular order, here are the 10 most common questions about the new module:
Q: What do you use to train the custom patent classifier? Title, abstract and claims? Just claims? Drawings?
A: The short answer is: the full specification, i.e. claims and description, currently. Abstracts are not included since they are redundant. Here’s some background: When any search is made in IPRally, the search is done in our databases of pre-processed knowledge graphs that represent the content in each patent. I say databases in plural as we have multiple databases of graphs – one database covers the full spec of the patent, another covers just the claims. When a "classifier robot" is trained, the AI uses the graphs of the patents (patent numbers) you have in your training set to identify the key attributes that these patents share. Currently, this is done by using the full spec graphs, but in a not too distant future you will most likely have more options to pick specific parts of the patents to use for the training.
Q: What data sources do you use in your platform?
A: We are not a data aggregator, and we are not aggregating any content ourselves. Our job is to develop technology that solves the problems we’re all facing every day when working on matters that require access to patent information. Hence, we buy our data from market leading data aggregators, such as IFI claims and others, and currently give access to roughly 100 million patents from 40+ jurisdictions, in high quality English translations. You can find more information about this here.
Q: User data is generated and used to train the custom classifiers, but do we also use this to enhance/train the general algorithms?
A: This is one of the most common questions we get, both with regards to the classifier and the general search algorithms. The short answer is no, we neither use the users’ labeled training data nor do we track or use any user behavior at any stage to train our general algorithms. The classifier you train is only accessible by you and your colleagues. We only train our public algorithms with public data. This is a deliberate choice as there are pros and cons with using user data for general training. On one hand it could potentially help and improve certain technology areas. On the other hand, though, it poses some ethical questions and it can also make the database biased in ways that we don’t want. So, no, no user data is used to train our algorithms.
Q: How does the custom patent classifier deal with complex hierarchical structures?
A: In its current, first version, the classifier does not support hierarchical structures per se. This does not mean that each level of a hierarchical taxonomy can’t be implemented as a specific tag, and that the classifier can’t learn and predict these classes. It just means that it cannot recognize them as part of a hierarchy, learn that hierarchy or display the information as part of a hierarchy. Yet. Alternatively, just the leaf classes of the hierarchy can be used as the labels. We are working on solving this in upcoming generations of the classifier, though. In the meantime, you can still train the classifier to learn the individual categories, and use things like naming conventions, color coding etc to structure the data.
Q: What are the general guidelines when it comes to training data? How much data is needed?
A: Along with the question about training of the public algorithms above, this is the most commonly asked question. And the answer can only be in the form of another famous question – how long is a piece of string? The answer is that it really depends. If you have a very granular internal taxonomy and operate in a technology area where there are large numbers of documents with very small differences between them, you will likely need more training data to teach the algorithm those nuances. If you have a taxonomy where it’s a lot easier to learn the difference between the categories, and the space is less intense, you will need less. The benefit with using Graph AI is that in any case, you will likely need substantially less training data to achieve good results compared to other methods. Many times a few dozen patents is enough to get started!
Q: What to do if there is no initial training data?
A: If you have internal training data – great! This will help you, and the more you have the more accurate the classifier will be. However, as mentioned above, one of the key benefits of using Graph AI to build classifiers is that it requires less training data than any other model. This means that you can easily build a training set in IPRally, and start with this. If you have a patent number, or a technical description etc, you can run a search based on these and just create a category based on the results you get. Once you have critical mass, you can create a classifier that you can continue to improve with new data as you go. Our just released automatic re-training mechanism ensures that the models learn in the background.
Q: How does continuous retraining and reclassification work?
A: Just like creating the classifier, the re-training, if you for example have added more documents to a category, just takes the press of a button. A second. And once the classifier has been retrained, it can be used to classify new, or re-classify known, datasets immediately. In a few weeks time you will also be able to retrain and improve the classifier directly through your confirmation and rejection of predicted tags.
Q: Is there a way to identify the confidence level of the predicted tags, and/or isolate the fringe cases or cases that fall just outside of the predictions?
A: Currently, the short answer is no, all predictions are made with a certain level of confidence. The user has the chance to change the sensitivity, i.e. the confidence level required. Predicting accuracy, or proximity, to a certain class is certainly doable though, and we will display the confidence shortly. This will allow our users to, once they know that the classifier does what it’s supposed to do, focus on the cases that are questionable and just accept the high confidence predictions.
Q: What if a single document belongs to multiple classes?
A: A single document can belong to multiple classes. This is not a problem, as this is the way it has to be, it is the reality. The patent will just have multiple tags applied to it when shown in IPRally, and it will appear when you run a search or filter by a tag.
Q: Is it possible to import and export classified data to and from other platforms?
A: Absolutely, data can be imported and exported into IPRally. If you have a set of patents that comes from a monitoring profile, a portfolio or something else that you currently have externally, you can easily import this into IPRally. Once this is done, you can apply the relevant classifier and predict the classes in this dataset. You can export either the predictions, or the confirmed classes once you have confirmed the predictions (one by one or in bulk). Both will appear in separate columns when you export the data into an Excel-file. You will see more and more benefits of doing all of this work within IPRally, though – watch this space! ☺
We hope that this answered some of the questions you had about the custom patent classifier, and where we are going with this. Do you have more questions? Ask! Do you want to try it out? Then sign up for a free trial here.